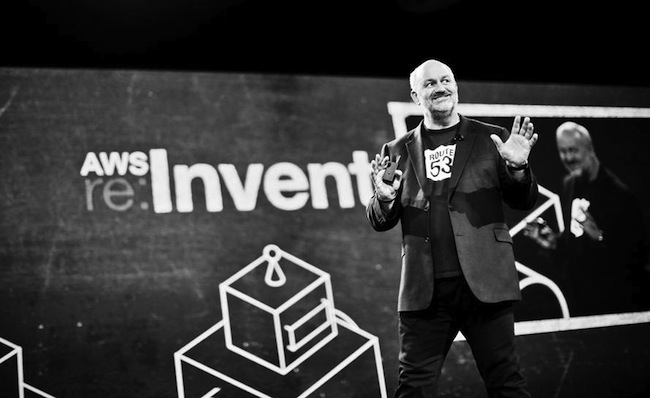
All Things Distributed
Werner Vogels on building scalable and robust distributed systems
Featured:
Recent Articles:
- Building with purpose: Stories from the Now Go Build CTO Fellows
- The next batch of CTO Fellows are reimagining healthcare
- Just make it scale: An Aurora DSQL story
- Alexa+ gets us a step closer to ambient interfaces
- In S3 simplicity is table stakes
- Thinking like a fox: A reading list for the future
- Tech predictions for 2025 and beyond
- Return of The Frugal Architect(s)
- Introducing the inaugural Now Go Build CTO Fellows
- AWS Lambda turns 10: A rare look at the doc that started it
- Empowering builders with the new AWS Asia Pacific (Malaysia) Region
- Continuous reinvention: A brief history of block storage at AWS
- Introducing Distill CLI: An efficient, Rust-powered tool for media summarization
- Hacking our way to better team meetings
- District heating: Using data centers to heat communities
- What I've been reading since re:Invent
- Tech predictions for 2024 and beyond
- Standing on the shoulders of giants: Colm on constant work
- Farewell EC2-Classic, it’s been swell
- Building and operating a pretty big storage system called S3